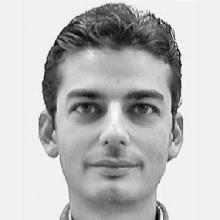
Research Activity
The approach envisages a fruitful synergy between applied and basic research. Specifically the research activities span three main directions, with a common ground where the reference technologies are probabilistic modeling and deep representation learning:
- The adoption of (latent factor) generative models in the areas of social media marketing, social network analysis and information diffusion and event forecasting. These models represent a challenge both from an application point of view (their adoption to model the propagation of information or in contexts where the data topology is complex is not trivial) and for the theoretical implications (the currently available learning techniques based on statistical inference are not adequate).
- Enhanced information filtering through integration of symbolic and sub-symbolic modeling. With a focus on how to build reliable and robust recommender systems, the activity consists in studying how to extend the current paradigms in two directions. On the one hand, we want to make recommendation systems capable of exploiting information structured in knowledge graphs. Knowledge graphs expand the amount of information available, thus strengthening the connections between the entities involved and providing more robust support (also in terms of interpretability) of the recommendation results. On the other hand, we want to combine user modeling and specification of domain constraints, in an approach that unifies reasoning and learning. In this respect, the current mathematical models can be considered “static”, being essentially adaptations of traditional supervised learning. However, we are interested in expressing dynamic modeling where predictions can be calibrated to contingent needs.
- Behavioral analysis of complex systems such as computer networks, social networks, industrial processes and sensor networks for environmental and energy monitoring. The goal is to detect and predict unexpected or anomalous events, in order to support the activation of effective countermeasures. The focus will be on two fundamental aspects. On the one hand, the need to guarantee adequate levels of efficiency in domains in which the quantities of data to be processed are massive and distributed in several peers. On the other hand, the ability of the models to adapt to changes and evolutions (concept drift) within the system being monitored.
Goals
The research group is focused on Behavior Computing and Analytics (BCA): that is, the study of efficient mathematical models for the analysis of entities (individuales, IoT/Mobile devices, smart objects…) interacting within complex environments. BCA represents an important topic in several contexts: user profiling and social computing, computational advertising and group decision-making, cybersecurity, opinion formation and dynamics, smart industry and society. The term “Behavior” here refers to a mathematical abstraction capable of summarizing, describing and predicting actions and reactions of entities in response to stimuli (pattern recognition), as well as possible deviances from their expected reactions (anomaly detection). The objective of the research group hence is to investigate mathematical and computational tools that allow to understand the structural and evolutive dynamics of the behavioral processes, in order to capture their foundational principles and predict both short and long term events and anomalies.
Application Fields
User Profiling & Computational Advertising (Recommender Systems), Industry 4.0 (predictive maintenance, process optimization, safety), Cybersecurity.
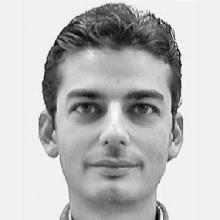
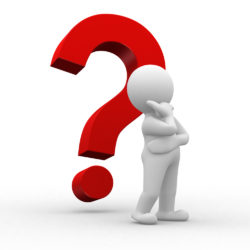
ERICA COPPOLILLO
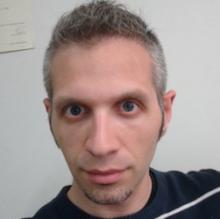
MASSIMO GUARASCIO
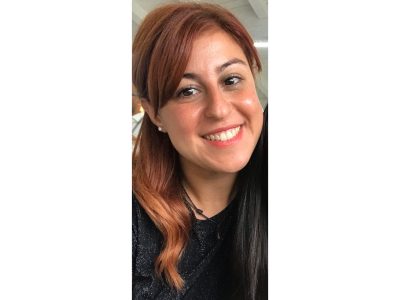
ANGELICA LIGUORI
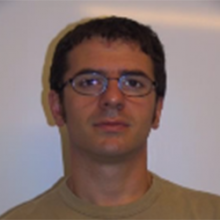
GIUSEPPE MANCO
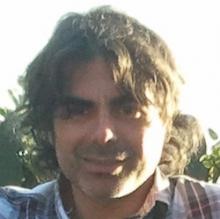
ELIO MASCIARI
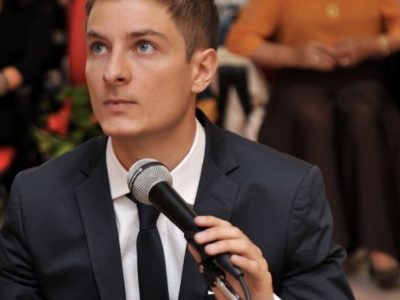
MARCO MINICI
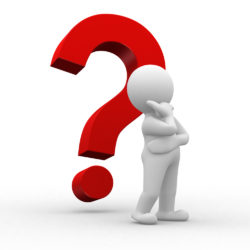
SIMONE MUNGARI
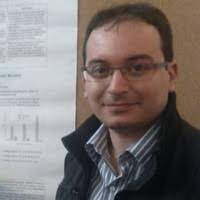
FRANCESCO SERGIO PISANI
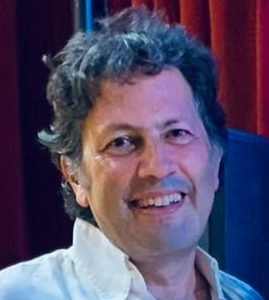
PAOLO ZICARI
- WHAM! – Watermarking Hazards and novel perspectives in Adversarial Machine learning
- SON – SecureOpenNets
- SERICS_Spoke_4: Operating Systems and Virtualization Security
- SERICS_Spoke_3: Attacks and Defences
- SERICS_Spoke_2: Disinformation and Fake News
- MIRFAK: Limiting MIsinformation spRead in online environments through multi-modal and cross- domain FAKe news detection
- iSafety – iSafety: Leveraging artificial intelligence techniques to improve occupational and process safety in the iron and steel industry
- HumanE-AI-Net – HumanE AI Network
- Humane AI – Toward AI Systems That Augment and Empower Humans by Understanding Us, our Society and the World
- CyberSec4Europe – Cyber Security Network of Competence Centres for Europe
- CATCH 4.0 : An intelligent Consumer – centric Approach To manage engagements, Contents & insigHt