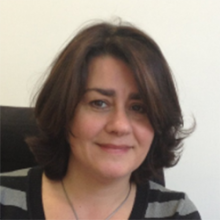
Research Activity
Self-Learning Systems, inspired by neuro-science and human-brain learning mechanisms, aim at learning solutions typically by means of try-and-error interactions with the surrounding environments or directly from collected data, rather than being manually pre-programmed.
Self-learning capabilities allow equipping complex systems with self-adaptation when deployed in dynamic and distributed environments. In detail, the research activities focus on the:
- development of novel self-learning methods by combining Inverse Reinforcement Learning and Forward Reinforcement Learning;
- definition of innovative self-learning hierarchical approaches, especially concerning the solution of multi-objective problems
- definition of self-learning distributed approaches via multi-agent systems
- development of methods and tools for the optimization of QoS parameters dynamically self-learned, rather than statically pre-defined
These methods exploit and integrate paradigms such as Reinforcement Learning, Inverse Reinforcement Learning, Multi-Agent Reinforcement Learning, Hierarchical Learning, Continuous Learning, Deep Learning.
Goals
The research objectives concern the definition of novel self-learning and self-adaptation methodologies for complex systems such as cyber-physical systems, multi-agent systems, robotic systems, and edge nodes, without relying on ad-hoc pre-coded knowledge.
Application Fields
Main application domains are: Healthcare, Industry 4.0, Robotics, Ambient Intelligence.
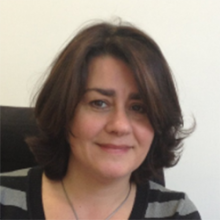
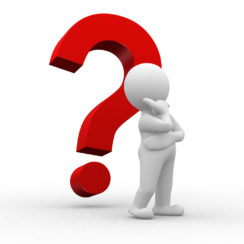
MARIA CATERINA MANNONE
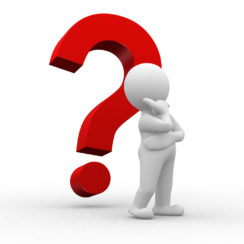
MICHELE PAOLETTI
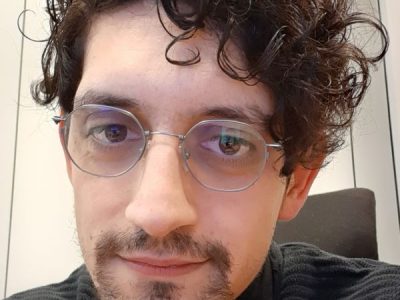
GIOVANNI PARAGLIOLA
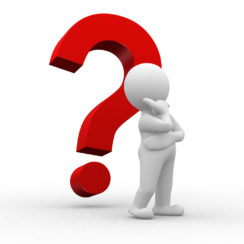
GIACOMO POTENZA
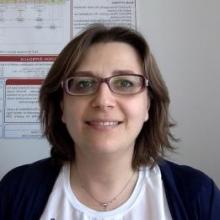
PATRIZIA RIBINO
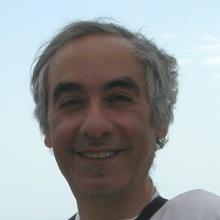
LUCA SERINO
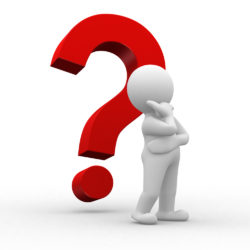
IHTESHAM SHAH
- ICU4COVID – CYBER-PHYSICAL INTENSIVE CARE MEDICAL SYSTEM FOR COVID-19
- DOSSIER – aDvanced mOnitoring SyStem wIth Enhanced security
- Development of an integrated radiomic and phenotypic system for the diagnosis, prognosis and personalized therapy of head and neck cancer therapy. Technological platform: eMORFORAD-Campania
- ASMARA – Applicazioni pilota post Direttiva 2010/65 in realtà portuali italiane della Suite MIELE a supporto delle Authority per ottimizzazione della inteRoperabilità nell’intermodalità dei flussi città-porto
- Age-It_Spoke_8: Interventions and technologies to reduce the burden of age-related diseases, disorders and disabilities